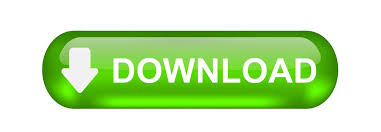
The study will provide useful guidelines on the effect of initial sample size and distribution on surrogate construction and subsequent optimization using LHS sampling plan. Each sample from LHS effectively represents each of N equal probability intervals of a cumulative density function. To achieve a better convergence, LHS evenly spreads out the samples to cover the whole range of the input domain. To build a surrogate model, it is recommended to use an initial sample size equal to 15 times the number of design variables. Latin Hypercube Sampling (LHS)¶ Latin hypercube sampling (LHS) is a pseudo-random, stratified sampling approach. Radial basis neural networks showed the best overall performance in global exploration characteristics as well as tendency to find the approximate optimal solution for the majority of tested problems. The surrogate models were analyzed in terms of global exploration (accuracy over the domain space) and local exploitation (ease of finding the global optimum point). The important findings are illustrated using Box-plots. The three surrogate models, namely, response surface approximation, Kriging, and radial basis neural networks were tested.

The analysis was carried out for two types of problems: (1) thermal-fluid design problems (optimizations of convergent–divergent micromixer coupled with pulsatile flow and bootshaped ribs), and (2) analytical test functions (six-hump camel back, Branin-Hoo, Hartman 3, and Hartman 6 functions).

Each factor is divided into nlevels, and the design points are arranged such that each level has exactly one point. An n-run and k-factor LHD is constructed as follows. The present study aimed at evaluating the performance characteristics of various surrogate models depending on the Latin hypercube sampling (LHS) procedure (sample size and spatial distribution) for a diverse set of optimization problems. Based on these two properties, a space-lling Latin hyper-cube design (LHD) is an appropriate and popular choice. In my previous post I’ve shown the difference between the uniform pseudo random and the quasi random number generators in the hyper-parameter optimization of machine learning.
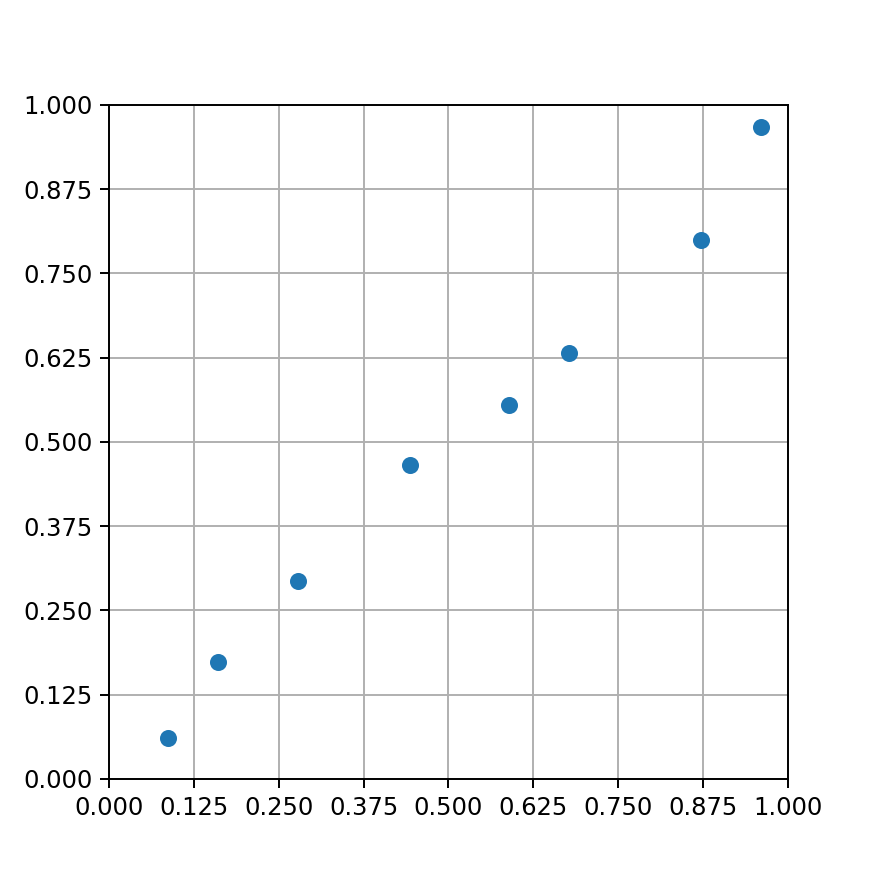
The exploration/exploitation properties of surrogate models depend on the size and distribution of design points in the chosen design space. Latin Hypercube Sampling (LHS) is another interesting way to generate near-random sequences with a very simple idea. Latin hypercube sampling is widely used design-of-experiment technique to select design points for simulation which are then used to construct a surrogate model.
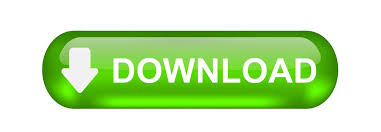